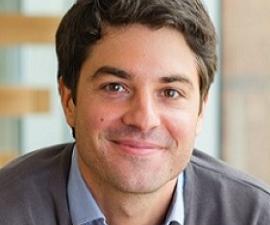
GRIFFIN SHUFELDT – APRIL 26, 2024
Ben Faber is a professor of Economics at UC Berkeley. His research focuses on development economics and has focused on countries in Africa, Asia, and the Americas. In Spring 2023, I had the opportunity to ask him about some of his research and background in economics.
Shufeldt: Before you did your PhD, you did your undergraduate degree in Geography and Economics, and your master’s was in Economic Geography. Could you tell us a bit about how you got into economics, why you’re interested in Economic Geography, and if you were always into Development Economics?
Faber: My main interest as an undergrad was studying economic development, and I had worked as a volunteer in several developing countries. I was interested in economic development and policy as well as environmental sustainability. It’s pretty common to have those things on your mind when you’re an undergrad. I came from Germany, from a German high school, and then, during my volunteering in Latin America, I interfaced with some international organizations and just asked them about career paths for work in economic development. They told me that a good option coming from Europe is to study in the UK, which has well-known universities.
I typed my application on a typewriter in Paraguay at the time, and I got into several UK universities. What convinced me to go to LSE over other universities was that the geography department had a geography and economics undergrad program that covered both environmental sustainability as well as development studies with economics. That, for me, was the selling point, having this broad, interdisciplinary setup. That’s how I got, not really into economics, but why I wanted to go into economic development and sustainability.
During undergrad, I got exposed to research. It became clear that I thought the more vigorous answers to the questions I cared about tended to come from economists. That sort of made me drift away from the broader social sciences and go into economics.
Shufeldt: So you were interested in development first and then figured out how to pursue that?
Faber: Exactly. I saw the side of development studies from the perspective of government and politics. Then, I saw development economics, and that was a very different animal with lots of statistics, lots of data analysis and lots of algebra. At first, the broader social sciences were more accessible, and you read more interesting articles; Over the years, I figured out that development economics, at least for my taste, required more statistics and math. But when I saw the output of it, like in the public talks and research seminars, I was more convinced by the answers.
Shufeldt: You mentioned that you volunteered in Latin America; Was this before undergrad?
Faber: Yeah, in Germany at the time, we had compulsory military service. I didn’t want to do the military service, so I did an international peace service, during which I worked for several social institutions in Chile, Bolivia, and Paraguay.
Shufeldt: Tell me about your paper on E-Commerce in China. You and co-authors wanted to find the effects of access to E-Commerce on households in rural Chinese villages. As stated in your paper, this experiment is reflective of the wide expansion of E-Commerce in China due to the government’s goal of reducing the rural-urban divide. After your experiment’s contrasting findings, what would you say about this policy’s potential to reduce the urban-rural divide in China, and in other developing economies?
Faber: Sometimes, you write papers that have big effects and are exciting, and sometimes, you write papers that sort of put cold water on something that has gotten a lot of hype. This paper falls into the latter category.
First of all, from a scientific point of view, we shouldn’t just publish papers that have surprising and big findings, right? Because if you only publish studies that have big and surprising results, then there’s this bias towards only publishing the part of the analysis that seems super promising. That’s sort of like a sample selection within studies. We randomized the rollout of e-commerce access to rural villages in China and worked with a big Chinese tech company to do this. All of the stakeholders, the government, the firm, and ourselves as researchers, from the existing case studies that have been around, were all very excited about the potential for consumers in villages to access e-commerce, for producers in villages, to start marketing to the big cities.
We followed up with our survey data and found that it only benefited certain households. Those households tended to be younger, richer, and more educated. Those households started spending a significant share of their budget on this new shopping option.
That’s sort of like voting with your feet. It’s what we call revealed preferences. So it’s good evidence that this shopping option added value to them in terms of their price index and consumption options, which you kind of expected, but it was much less uptake than I think any of these stakeholders had expected.
A minority of rural citizens started purchasing stuff. We found nothing of the big hype about this policy that came from several case studies, where a village was transformed into a production hub and started specializing in making one niche product and marketing it all over China, exporting it to the rest of the world. Several past case studies examined these e-commerce villages that had transformed into production hubs. But, we found nothing on the income side. We found nothing on the production side.
I think this is a meaningful finding, in those studies that say that e-commerce transformed the village, it doesn’t mean that e-commerce didn’t transform the village in that case. It’s just when you estimate the effect in a more representative cross-section of villages in China, and you randomize the rollout, the transformative effect of e-commerce access doesn’t seem to hold in a sample of villages that were chosen by the government and the firm for this rollout.
Shufeldt: So it’s not a huge, broad thing. There are some positive effects in some populations, in some rural villages.
Faber: The interesting follow-up question that comes up out of this study is the following: why is it the case that e-commerce has evidently transformed production in certain rural marketplaces that you see all over the map?
In China, there are more than a thousand villages that have been branded e-commerce villages because everything they produce gets sold online. They’ve been very successful in creating income that way and transforming the rural economy. Why, on one hand, do you see these very successful case studies popping up all over China, and at the same time, when you do a randomized controlled trial evaluation over positively selected villages, it doesn’t seem to hold. What makes those transformed markets special?
Often you have these anecdotes, like an entrepreneur came back to his hometown and started something, he or she had connections to the cities and financing. You hear these are all anecdotes, but often it started with a person who had a network, and then around that person, there was a whole ecosystem flourishing.
Shufeldt: What do you think about the role of satellite imagery in development economics? Do you think that it’s going to become more widely used? Is it at an appropriate level of usage right now?
Faber: The biggest advantage of satellite data is that it has existed for the last four decades at a pretty high resolution. The resolution always gets better and better. That’s great because many, especially poor countries around the world, don’t have good administrative data on, for example, farm productivity. Satellite data is basically a couple of data points for a pixel on the globe. What you see is a data value within a wavelength. You get a bunch of data points for different wavelength bandwidths of light that get emitted.
This data can, often using machine learning, indicate what was grown on a pixel and how much was growing on the pixel. If you grow corn or wheat, you can train an algorithm on what the combination of data points across different bands of light are, if you’re looking at a cornfield that has a yield of X or Y.
You can also do it with nighttime lights. It becomes like a proxy measurement of economic activity, either in agriculture or using electricity, that you have available over time and without any data restrictions.
Sudan does not have good census data on agriculture. However, that doesn’t mean you simply don’t have data on Sudanese agriculture. You have it for pixels across the globe. So for measurements, it’s very rich, and it’s very informative, especially in poor data environments.
The downside of satellite data is that you can only see those reflections of light in different bands, and that’s all you are ever going to get. Often we use this with machine learning to say, this village seems to be richer than another village. Imagine you had administrative data on village income for a training data set. You have satellite data for this village, all the pixels of the village, and you have the real GDP per capita of this village. Then, you can use machine learning to train an algorithm that converts the satellite data and makes it predict real GDP for the village. Taking it even further, you can use that trained machine learning algorithm to say, now I only have satellite data, so now tell me what the real GDP of this village is where I don’t have the administrative data.
That’s the frontier of this research, but you have to be careful about this. You don’t really understand what in those pixels indicates that this village in the administrative data was rich or poor.
So imagine what the algorithm really picks up is whether you have a certain type of roofing in this setting, and the roofing goes to being made of metal from mud. The algorithm, using the satellite data would pick up that the roofs are different, going from mud to metal. In this particular setting, in this particular cross-section, that may be very informative in this particular country setting.
Now imagine you use that same trained algorithm to predict growth rates going forward in the same country—imagine that nothing really changes to roofs over time. If you don’t really understand why these particular values of reflected light happen to predict real income well, but then use that blindly to say this is the effect of a policy of unconditional cash transfers, it becomes borderline dangerous. It’s very useful, but you have to be careful.
Shufeldt: So it seems like there’s an exaggerated external validity concern.
Faber: Yeah, it’s a black box. You train this thing intensely, and it predicts really well. It predicts a sample in the data that you have, but then you go and take this across the globe. Chinese roofs may be very different from Sudanese roofs. All of a sudden, you predict something very different, and you don’t know why and don’t have the administrative data.
Shufeldt: Regarding one of your working papers, scaling agricultural policy interventions, what are some of the core questions you and your co-authors wanted to answer, and what are the potential policy implications for Uganda and other sub-Saharan countries?
Faber: What has happened over the last few decades is that a lot of policy analysis got transformed by the use of randomized control trials to estimate the effect of a policy for the government in a really credible way. Consider if the government wanted to roll out a subsidy for modern input use in agriculture. Before they roll this out nationally, they’ll work with the World Bank or researchers, and say, “Let’s do a randomized control trial so that we know what’s going to happen to farmer income and if this is a good policy for us to consider.”
Usually, such a policy is evaluated on a smaller scale. You’re interested in estimating the first small rollout of this intervention to see if it’s promising before it’s rolled out to a broader segment of the population.
This is related to the caveats that you have in RCTs. In a small-scale RCT, what you’re going to estimate is the effect of a policy on the outcomes of farmers in this example, holding their output prices and their input prices pretty constant because usually, the randomized control trial where you treat 200 farmers there and don’t treat a different set of 200 farmers is not at a scale that would affect the economy as a whole in terms of decreasing the price of crops.
But if it decreases the price of crops, then that’s going to eat into the farmers’ incomes. Whereas, if you do this in a small-scale RCT, crop prices are unaffected. You’re giving them a subsidy for an input, so they can sell at the same output price that they had before and save on input costs.
Maybe the income effect of that is stronger than if you gave that to every farmer in the region, where the output prices could come down, and maybe the wages of their workers go up. One way to get at that is, of course, experimentally, but that gets very expensive, and it sort of goes against this like, “Let’s test this first before we roll this out.”
So, what we do is propose a combination of the effects that you’re finding in the small-scale RCT, with a theoretical structure in which you can plug in those effects and then predict what would happen, according to economic theory, if you saturated the entire country with that policy.
And then there’s uncertainty. The model makes assumptions for us to be able to solve that, but at least you get an indication of the direction where it would go and the regions where it would differ and the distribution implications and in which direction they would go.
The idea behind that paper is that we propose a sort of toolbox where you can use the results from a small-scale RCT, plug them into a theoretical structure, and then ask the computer, “Okay, now please solve for the welfare consequences of this policy,” using the parameters that you’ve estimated in the small-scale RCT.
Shufeldt: Is that model specific to the previous RCT that was run, or could it be applied elsewhere?
Faber: So that’s the tension, right? Ideally you want to make that model as specific as possible to the country context. So the model is geared towards being valid in agricultural settings. Agricultural crops have a number of features that are very different from electronics.
The good thing is that it’s not just a theoretical structure where you plug in the treatment effects of your small-scale RCT. You also do something that we call calibrating a model, that is, you tell the model how many households live in which markets. We do this for Uganda. You could have done this for Kenya, and it would’ve been a very different geography in terms of the number of households, in terms of where those households are living and how those markets are connected.
Basically, you can be specific to the context by telling the model where the households live, how rich they are, what they’re growing, and what the trading costs are between them. Each piece of information is going to adapt the model to a different economic geography. You can adapt it to the setting just by populating the model in a different way.
Shufeldt: What’s one piece of advice you would give to a student interested in international development?
Faber: I would tell the student to try to go to research seminars, which are all open in Berkeley. There’s development talks at Haas, there’s development talks at ARE, there’s development talks in the Economics Department, there’s development talks at the Goldman school, there’s development talks at the East Asian research center. There are talks about economic development all over campus, and then there are public talks which tend to be like, “I have a new book”. Then there are research seminars where professors from other universities come and present their recent research.
Berkeley is a great university, which means that you will see speakers who have exciting new papers. So by sitting in the room, you will learn about the forefront of research into poverty alleviation. Also across different fields, you will learn about how answers are being crafted to policy questions in real time.
You also see different methods. Some methods are more qualitative. They interview lots of people who live in the villages. They really have a deep qualitative understanding of the meaning of things that are going on. Others are more quantitative, they just have a data set and run regressions, and you can see what clicks better with you if you find it convincing.
The other thing is, in your summers and off-time, try to get internships or working experiences for organizations that work in international economic development. Those could be summer internships at the World Bank, NGOs in the Bay Area, startups that want to make a difference and also turn a profit, or a tech foundation in the Bay Area. They often have an angle for international development.
The truth of it is that poverty is still there. We’ve made lots of strides against it, but it’s not like we have the best answers yet, despite all the evidence that we have. Basically what happened to me is I worked in different fields in economic development, and I found some of them as doing something really sensible that I believed in, and some of them as pushing paper and not doing something I believed in.
People can have very different preferences. Some people may find that working at an orphanage in some developing countries is the most meaningful thing you can do. Forget about publishing economic papers. Forget about raising income on a global scale.
Expose yourself to both the research and practitioner sides of things. Even if you decide against international economic development after you’ve done that, these experiences are valuable for anything else you want to do.
So that’s the good thing, right? It’s not like you’re doing something super specific to the point that nobody else will ever talk to you again if you don’t go into that field. You know, global economic development is such a broad and connected question with many sets of actors in it that I don’t think you can waste your time by doing it.
Featured Image Source: UC Berkeley
Disclaimer: The views published in this journal are those of the individual authors or speakers and do not necessarily reflect the position or policy of Berkeley Economic Review staff, the Undergraduate Economics Association, the UC Berkeley Economics Department and faculty, or the University of California, Berkeley in general.