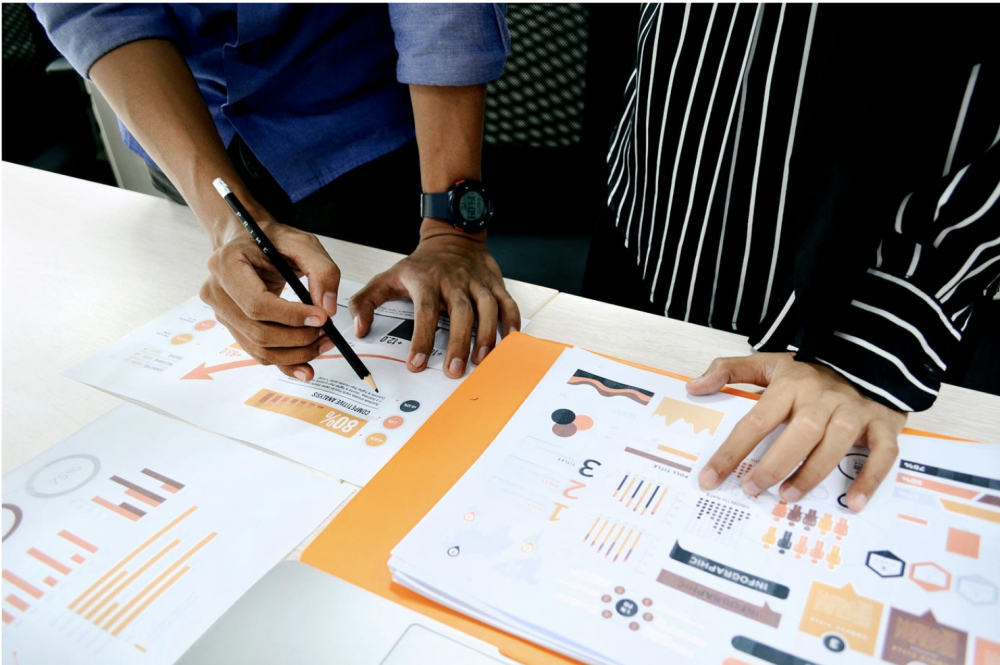
ANEESH KUDRIMOTI- MARCH 22, 2023
EDITOR: AAYUSHI SINGH
ABSTRACT
Collaboration is a critical component of productivity and innovation in economics research. Despite its importance, there exists little to no literature examining how lockdown measures have impacted collaborative practices in the field. In this study, I aim to investigate the effects of stay-at-home orders implemented during the COVID-19 pandemic on coauthorship networks in economics research. More specifically, I look to examine the extent to which a range of pandemic-related factors have influenced the average distance between coauthors: a proxy for collaboration. These factors include the growth of virtual communication platforms, heightened gender inequality, challenges in conducting fieldwork, and the incentive to collaborate with researchers from different universities.A stratified random sample of 200 NBER working papers issued before and after the implementation of stay-at-home orders was collected and analyzed. A series of regression models were then used to examine the impact of the aforementioned factors across the pandemic. However, while point estimates suggest economically important heterogeneity in the pandemic’s effects on coauthor proximity, estimates in the random sample were not statistically significant, thus motivating further investigation.
(1) Introduction
(1.1) Background/Motivation of Study:
A sizable body of research has been conducted on the negative implications of the pandemic on productivity, innovation, and mental health in academic research. This is not surprising as initial lockdown restrictions forced many research institutions to shut down or reduce their operations, resulting in delays in existing research and a sudden shift to remote work. However, little research has been done on the flip-side of this phenomenon. Running parallel to the perceived negative effects of the pandemic on research is the rapid growth of video-conferencing platforms like Zoom. To put this into perspective, between 2019 and 2020, Zoom meeting participants increased nearly 300% (Statista, 2022). The growth of video-conferencing certainly indicates that human beings have adapted to the challenges of the pandemic by embracing new technologies in order to stay connected and continue working despite social distancing measures. In relation to academic research, however, several questions arise:
(1) Has the shift towards virtual communication restored collaboration to pre-pandemic levels, or has it enabled even greater collaboration and productivity amid the ability to overcome geographic barriers?
(2) Have stay-at-home orders and a transition towards the use of virtual communication for work incentivized researchers to collaborate outside their affiliated university or institution?
(3) To what extent do factors such as gender inequity and limitations in conducting in-person fieldwork mitigate this potential increase in collaboration?
In this paper, I seek to address these questions, with a focus on collaboration networks in economics research. I chose to focus on this field in particular because it frequently involves collaboration across academic institutions and countries, making it an ideal framework to examine the effects of social-distancing measures on collaboration networks amid geographical barriers. My initial hypothesis is that collaboration in Economics research as a whole has transformed after the pandemic due in part to the growth of virtual communication platforms, which have allowed researchers to expand coauthorship networks. This is because geographic barriers no longer present an issue and because researchers no longer experience the same incentive to work within their school or workplace. Moreover, I believe that gender inequality and complications in conducting fieldwork will play in part in mitigating this effect through the delay or cancellation of projects, hence decreasing overall productivity and collaboration. I am curious, however, to examine whether these adverse effects are large enough to completely nullify any positive effects.
(1.2) Literature Review:
To explore the research questions defined above as well as my initial hypothesis, I want to preface some of the existing literature in the field. Although the authors in the literature mentioned primarily examine the effect of lockdown measures on productivity in academic research, rather than collaboration, their research. still offers valuable insight into the multiple ways the pandemic has shaped research and impacted researchers. This literature review is structured into three sections: productivity in the sciences, productivity in economics research, and gender disparities in research—all in relation to the pandemic. In each section, I will summarize several relevant findings as well as limitations that my research seeks to address.
(1.2.1) An overview of literature on productivity in S.T.E.M research
Much of the research done on fields outside of economics—largely in S.T.E.M fields—explores two contrasting effects of the pandemic on research output. On one end, the pandemic has disrupted the ability to conduct lab-based scientific work, which is essential to advancing research in the sciences. As one paper published in Springer Nature highlights, the closure of labs and lab-based scientific research activities during initial lockdown measures has not only hindered research progress and projects, but also created additional pressures related to indirect costs (Radecki & Schonfield, 2020). These indirect costs include universities’ pressure to secure stable revenue streams due to diminishing flexibility in federal funding, and the challenge of supporting existing research due to the lack of academic instruction that often subsidizes research. As outlined in a paper published by Springer Nature, the indefinite timeline for continuing research, coupled with unstable funding sources, has taken a toll on scientists. Postgraduate and early career researchers, in particular, have been deprived of networking and publishing opportunities (Fosci et.al, 2020). Although the literature mentioned reinforces the pandemic’s negative effects on research output, an article published by the NIH suggests that the pandemic has led to a massive influx of scientific publications on COVID-19, which currently accounts for 10-20% of current biomedical investigation (Harper et.al, 2020). Thus, output in the sciences has paradoxically both been drastically limited by the pandemic and expanded due to the necessity of medical research to limit the spread of Covid-19. The literature provides valuable insights into factors such as limited capabilities and opportunities for researchers, funding issues, and general uncertainty that have affected research productivity in academia and, as a result, collaboration during the pandemic. Moreover, it further displays the push-pull effect of various factors on academic research that make it difficult to tell whether productivity and collaboration in academic research as a whole is trending upwards or downwards in recent years. While differences in collaboration amongst early-career researchers and postgraduates are not explicitly examined in this paper, this interaction may have potentially impacted the estimated coefficients in the initial model I constructed (see 2.2). The literature has mainly relied on ethnographic evidence to examine factors affecting productivity and collaboration. In this paper, I apply a causal inference approach to build on the existing ethnographic evidence.
(1.2.2) An overview of literature on productivity in economics research
In a recent study published in the Oxford Academic, Samuel Kruger, a professor of Finance at the University of Texas, Austin, points out how it is difficult to predict whether stay-at-home orders will have a positive or negative effect on economics research. On one hand, Kruger notes that economics research traditionally heavily relies on in-person seminars, conferences, and informal office conversations, many of which came to a halt in March 2020. On the other hand, he describes how Covid-related challenges present new opportunities for economics research particularly in fields like healthcare and public economics. This positive effect is further increased due to how economics research generally involves the use of existing datasets, as opposed to data collected in a lab or through fieldwork, which makes it an ideal candidate for efficient teleworking. To accurately measure the direction of this change, Kruger examined a set of working papers posted on the Social Science Research Network (SSRN) by faculty at top-50 U.S. economics and finance departments. To quantify production, they measured the frequency at which papers were posted to the SSRN by faculty, and used a difference-in-differences model to determine a statistically-significant change in research output before and after the pandemic. The key finding is that following the onset of COVID-19, research production in economics and finance (measured by the posting of working papers) increased by 29%. This figure shows the resilience and potential evolution of economics research in the face of the pandemic, which is somewhat of a contrast to the S.T.E.M. fields discussed above.
The most relevant finding from his paper was an “increased reliance on past coauthorship networks” within faculty and “larger production gains for authors that are more central to the network”. This finding is useful in that it partially addresses my first research question: coauthorship networks may have not expanded drastically after the pandemic. Yet, this conclusion does not provide insight into how collaboration dynamics may have shifted during the pandemic. In other words, it does not detail whether researchers in the study potentially overcome geographical barriers to maintain their co-author networks, which is especially relevant given the unique circumstances of remote work and reduced in-person interactions. In my paper, I mainly focus on incorporating this potential shift in collaboration dynamics by defining a metric that can measure collaboration in relation to geographical barriers.
It is also important to acknowledge the potential negative impact of the pandemic on subfields within economics that require extensive fieldwork, such as development economics. While empirical work in economics typically relies on existing datasets, development economics has a rich history of conducting field research. Research in this sub field often involves taking structured visits to the field to better understand the economic environment being studied and to clarify aspects of large-scale data sets through sampling and survey methods (Udry, 2003). With this in mind, it would make sense that an inability to conduct fieldwork would hamper productivity in the field as researchers are no longer able to engage in these crucial data collection processes. This has certainly been the case for researchers in other social sciences, like political science and psychology, that often make use of fieldwork as a component of their research. For example, Aidan Motliff, a PHD Candidate in Political Science at MIT, describes how her work on political violence in India often relies on the ability to conduct interviews in-person and the support of her Indian colleagues, both of which have been put on halt due to lockdown measures (Krause et.al, 2021). Dr. Tapiwa Madimu, an economics historian at Rhodes University, states that researchers face a difficult decision: to cancel or postpone projects or to continue despite potential health risks (Madimu, 2021). Overall, ethnographic evidence suggests a negative impact on collaboration in these fields. In this paper, I aim to corroborate this existing evidence through a more quantitative approach. This approach may not only reveal effects within development economics but also shed light on other subfields that heavily rely on fieldwork.
(1.2.3) An overview of gender disparities in academic research
A common theme of the literature I examined was a striking gender disparity in research production during the pandemic. In the NIH journal article mentioned in (1.2.1), the authors discuss how early analysis on publications in-and outside of scientific research have shown that “female academics are publishing less and starting fewer research projects than their male peers.” The authors specifically point to the increased familial and childcare responsibilities that women are facing during the pandemic due to having to work from home. In a related article published in The Guardian, the authors interview several female academics in the UK to gain insight into this potential gender gap. One female academic explains this disparity in terms of the historic wage gap, saying “because she earns less, and can be more flexible about when she works, the bulk of the childcare falls to her.” Both articles provide some qualitative evidence on gender disparity in research production through anecdotal evidence from female academics, but are limited in that they don’t show a statistically significant difference between production across males and females. In the paper mentioned in (1.2.2), Kruger incorporates this perceived difference into their modeling to limit potential noise in his regression model. The key finding from the paper was that “women between the age of 35 and 49 experienced a production increase that is 0.31 papers per year smaller than men in the same age group, a difference that is statistically significant at the 1% level.” In addition, researchers found a mean 6% increase for women aged 35–49 compared to a mean 32% increase for men aged 35–49 before and after the pandemic. Both of these statistics, again show a sizable and statistically significant change in production sectioning on gender. Overall, this literature provides sufficient evidence that gender disparities are likely associated with decreased research productivity. However, the anecdotal evidence detailed in the NIH article along with the empirical evidence presented by Kruger, are not sufficient enough to draw conclusions about changes in collaboration practices within economics. In this paper, I seek to address this shortcoming by utilizing a regression model with an interaction term to explain gender disparities across the pandemic. I aim to establish a causal relationship between pandemic-induced gender inequity and collaboration, and how this has potentially masked the neutral/positive trend in economics productivity described by Krueger.
(2) Data Collection and Research Design
(2.1) Data Cleaning and Collection
My paper primarily relies on the metadata of the NBER working paper series, which contains details such as titles, coauthors, abstracts, and dates of NBER working papers from 1973 to 2023, and is publicly accessible. NBER working papers are particularly well-suited for this study due to three key reasons: (1) they are working papers, which means the actual collaboration necessary for the paper occurred close to their publication date, rather than years earlier, (2) they are authored by at least one NBER affiliate, thereby ensuring their credibility, and (3) all the papers are related to economics, which is the main focus of this research. I was able to access this data thanks to a blog article written by economist Alex Albright. This article focuses on publication metadata of NBER working papers and provides some intriguing descriptive analysis of this data (Albright, 2021).
Given that nearly 1200 working papers are published in the NBER working series every year, the data set contains nearly 33,000 entries of 41 variables. As such, I seeked to clean the raw data in R-Studio to obtain the necessary information related to my overarching research question.
As mentioned in Section 1.1, I am primarily looking to see whether the introduction of lockdown measures, and thus a rise in virtual communication, has brought collaboration back to pre-pandemic levels or possibly facilitated even greater collaboration and productivity. I thus defined a metric that could quantify both a change in collaboration and the geographical barriers introduced by lockdown measures — the average pairwise distance between coauthors. Formally, this measure is:
In the measure above, c1, c2, c3 represents each coauthor on a working paper. I filtered the data in the raw dataset to include only papers with exactly 3 coauthors, so as to simplify calculations for this metric. Also, the ordering of each coauthor is essentially arbitrary in the equation, and so while the first coauthor listed in each paper has made the most contribution to that paper, it is not of relevance when calculating the distance between coauthors. The exact calculation for the pairwise distance between a particular set of coauthors is the Haversine distance formula, which computes the distance between latitude-longitude pairs for a particular location while accounting for the curvature of the earth. The use of this formula was to ensure calculations were as accurate as possible.
The potential implications of this metric are twofold: One possible implication is that due to lockdown measures, NBER affiliates may no longer be able to work with colleagues in close proximity. As a result, their communication networks may expand outside of the university where they work and potentially reach other universities. This is because the cost of communication with a researcher at their university becomes virtually equal to the cost of communicating with a researcher in any other location, as proximity is no longer a factor. This would be reflected as a higher expected distance between co authors after lockdown measures are instituted. On the other hand, there are factors mentioned in the literature—such as gender disparities in pandemic-era familial and childcare responsibilities, diminished in-person interactions at events such as conferences, and a reduced ability to conduct research in fieldwork-driven subfields —that have the potential to actually diminish collaboration as a whole. This would be reflected as a lower expected distance between coauthors after the pandemic. Thus, this metric accounts for both possibilities and provides a strong proxy for collaboration.
To obtain the data to compute this metric, I cleaned the raw data set to include the name of each paper in the data set, a set of coauthors (each in their own column), and the issue date split into three columns containing year, month, and date. Once I did this, I sectioned off the data set into a set of papers published between 2016 and 2019 and a set of papers published between 2019 and 2023. I then randomly selected a set of 100 papers within each group. Unfortunately, the coauthor affiliation and coauthor gender data was not contained in the dataset I was working with, nor could I find this data in any other publicly available source. I thus had to manually enter the affiliation and gender of each coauthor, as well as the JEL categorization of that paper for each set. The JEL Classification is a comprehensive categorization of fields that nearly all papers fall under (e.g. Category O corresponds to Economic Development, Category R corresponds to Urban Economics, etc.) and is noted in the bibliography. All of this metadata could be found on the first or second page of each working paper as such:
Once I recorded the university of each coauthor, I computed the average pairwise distance between each coauthor in kilometers using the metric defined above. I encoded whether or not a paper had at least one female coauthor as binary variable gender (1 = has at least one female coauthor, 0 = no female coauthors). I encoded the field of each paper as a set of binary variables corresponding to its JEL classification. Since papers typically fell into multiple JEL categories, I usually chose the most common subcategory listed or in the case there were multiple unique categories, I chose the one that was most fitting based on what I could gather from the abstract. In my analysis, while I encoded the paper for nearly all JEL categories, I ended up only examining whether or not a paper fell under development economics. This is because I chose development economics to encompass the effects of fieldwork-heavy subfields on collaboration based on the literature mentioned above. I also converted the year column to a binary variable pandemic which represents whether the paper was written before or after April 2020 (1 = after, 0 = before), as this is typically when lockdown measures were put into place (Link) I then merged the two separated data sets back together into one dataset so I could conduct regression analysis. Here is a look at the first few entries of the cleaned data set used in my analysis:
(2.2) Research Design + Modeling
To reiterate, the main purpose of this study is to answer the following questions:
(1) Has the shift towards virtual communication restored collaboration to pre-pandemic levels, or has it enabled even greater collaboration and productivity amid the ability to overcome geographic barriers?
(2) Have stay-at-home orders and a transition towards the use of virtual communication for work incentivized researchers to collaborate outside their affiliated university or institution?
(3) To what extent do factors such as gender inequity and limitations in conducting in-person fieldwork mitigate this potential increase in collaboration?
To gain some initial insight into the first question, I constructed a simple regression of the average distance between co authors on pandemic.
The interpretation of the intercept term ꞵ0 is the average distance between coauthors before the pandemic. The interpretation of the coefficient for pandemic, ꞵ1, is the expected change in the average distance between coauthors after the pandemic. Since pandemic is a binary variable, this is essentially identical to conducting a t-test for difference in means. The regression estimates from this model are obviously subject to omitted variable bias considering factors mentioned in the literature such as gender disparities, field, status of coauthors, funding, etc.. However, the ‘biasedness’ of the ꞵ1 coefficient is actually useful in that it can demonstrate the degree to which positive effects of the pandemic on collaboration, such as the increased use of virtual communication platforms in collaborative practices, has been offset by the aforementioned factors. Isolating the degree to which gender disparities or the ability to collaborate have affected collaboration across the pandemic, can not be extrapolated from this regression. I thus construct two additional regressions to capture these effects.
In the above regression, I used an interaction term to estimate the causal effect of gender disparities on collaboration after the institution of lockdown measures. ꞵ0, ꞵ1 and,ꞵ2 serve as control variables. The interpretation of ꞵ0 is the expected distance between male coauthors before the pandemic. The interpretation of ꞵ1 is the change in the expected distance between male coauthors after the pandemic. The interpretation of ꞵ2 is the difference in the average distance between female and male coauthors before the pandemic. Finally, ꞵ3 is the coefficient of the interaction term of gender and pandemic. This indicator variable is essentially turned on for papers with at least one female coauthor that have been produced after the pandemic and thus measures the effect of gender disparities across the pandemic. I expect the coefficient for ꞵ3 to be large and negative as the gender disparities have become larger after the pandemic. I would also expect the coefficients of the male control variables to be positive and for ꞵ2 to be negative but somewhat smaller than ꞵ3. If ꞵ2 was larger than or close to equivalent to ꞵ3, it would indicate that gender disparities are largely pre-existing and independent of whether or not a paper was published after the pandemic.
In the final regression, I use an interaction term to capture the causal effect of complications in fieldwork due to the pandemic on collaboration. The underlying theory behind including this interaction term is that due to the pandemic, NBER affiliates whose research is in development will no longer be able to travel to field sites, and so authors from local universities where fieldwork is conducted will no longer appear in the list of coauthors for a particular paper in development. This phenomenon would be reflected by an decrease in the average distance between coauthors after the pandemic. As a reminder, I am treating papers classified under development economics as an indicator for field-specific effects. ꞵ0 represents the average distance between coauthors before the pandemic for fields outside of development economics, which I treat as fields that do not typically involve a great deal of fieldwork. ꞵ1 represents the difference in the average distance between coauthors after the pandemic for fields outside of development economics. ꞵ2 represents the difference in the average distance between coauthors within and outside of development economics before the pandemic. ꞵ3 is the main focus of this model as it is a causal estimator for the effect of the pandemic on collaboration within development economics.
(3) Results and Discussion
(3.1) Results
The results of the series of regressions are detailed below:
(3.2) Discussion of Results
For the first model, we can interpret the coefficient on pandemic (ꞵ1) as the expected decrease in the average distance between coauthors is approximately 193 km after the pandemic. In other words, this corresponds to around a 7.6% decrease in collaboration after the pandemic. This likely signals that factors like the negative impact of gender disparities and complications in the ability to conduct fieldwork have outweighed positive factors mentioned in the literature, such as innovation generated from Covid-19 related issues or the ability to continue research in subfields that typically don’t involve heavy amounts of fieldwork. Note that the t-stat for this coefficient is 0.49, which makes it difficult to generate conclusions from the results. This problem is generated by the high standard error in the coefficients, which can be attributed to the sample size of 200 in the study. For the second model, the estimated slope coefficient on the interaction term is positive. This is contrary to my hypothesis, as my intuition was that gender inequity would become more pronounced after the pandemic relative to before the pandemic. Although, the slope coefficient on gender (ꞵ2) is already relatively large and negative, which could potentially indicate existing gender differences in publications were already an issue before the pandemic and thus while gender disparities may have appeared to gone down, they are still large in effect size. To put this into perspective, papers coauthored by at least one female researcher after lockdown measures were instituted showed a predicted decrease in the average distance between coauthors by 241 km, compared to research conducted solely by male coauthors before the pandemic. This amounts to around a 10% decrease in collaboration for female coauthors relative to their male peers. Again, results must be taken with a grain of salt as the coefficients on these models as the test statistic for our interaction term is 0.81 and 0.71 for gender, neither of which meet the threshold for statistical significance at the 5% level (1.96). For the third model, the estimate of the slope coefficient on the interaction term (ꞵ3) is negative, which aligns with our hypothesis that collaboration as a whole within development economics will have decreased after the pandemic. We can also examine the partial effect of the pandemic on Economics as a whole, which can be done by taking the partial derivative with respect to the variable pandemic of our population regression function and plugging in our coefficient estimates. Taking our partial derivative gives us the partial effect of ꞵ1 + ꞵ3 * (development). Since both are large and negative in effect size (-248 and -343 km respectively), there is a diminishing effect on collaboration for fields outside of development after the pandemic, and an even more severe effect for research within development economics. This corresponds to a 11 percent decrease in collaboration for non-fieldwork heavy subfields of Economics and a nearly 30 percent decrease for fields involving a great deal of fieldwork. This sizable decrease in collaboration confirms my hypothesis that the inability to conduct fieldwork diminishes collaboration in Economics both within development economics and in other subfields. It likely appears that neither a shift towards virtual communication nor an incentive to collaborate with research from other universities was enough to promote increased collaboration, even in fields where work revolves around existing data. Note that statistical significance of our estimates is a slight issue here as only our coefficient for development is highly statistically significant. The interpretation of this coefficient is still pretty interesting as its strong, positive effect size of around (2940 km) implies that before the pandemic, collaboration was much more integral to development economics than it was to other fields.
To improve this study, a larger sample size is needed. As not all the paper metadata I needed was available on the NBER website, REPEC, EconLit or any other database, it became difficult to manually collect the metadata needed for a sufficient sample size. Ideally, a random sample of around 1000 would be needed to produce more statistically significant estimates on the slope coefficients. To accomplish this, it would be up to NBER to update coauthor data available, however this is understandably a privacy issue. Another alternative would be a web scraping algorithm that is authorized by the databases mentioned above. With regards to the model, it also may be beneficial to test non-linear models to account for outliers or to simply make interpretations of the coefficient more comprehensible. For example, a log transformation of avgdistancebetweencoauthors, would make the slope coefficients refer to a percent change in the outcome variable as a 1% increase in distance between coauthors is much easier to interpret than a 1000km increase in the distance between coauthors. Overall, a more concrete understanding of pandemic effects requires a larger sample size and perhaps some fine-tuning for our model.
(4) Conclusion
In this study, I sought to determine potential causal effects of lockdown measures instituted during the Covid-19 pandemic and collaboration in economics Research. To investigate this, I utilized a publicly available record of NBER working papers from 1973 to present-day. After dividing up the set of working papers into those published between 2016 and 2019 and 2019 and 2023 and taking a random sample of 10- papers within each group, I recorded data on the universities, gender, field, and distance between coauthors for each individual paper. I then utilized a series of regressions to determine the causal effects of lockdown measures accounting for potential gender and field-related differences in research collaboration. While point estimates suggest economically important heterogeneity in the pandemic’s effects on co-author proximity, a larger sample size is needed to obtain statistically significant results.
Featured Image Source: Why is Big Data Important in Our Life and Business
Disclaimer: The views published in this journal are those of the individual authors or speakers and do not necessarily reflect the position or policy of Berkeley Economic Review staff, the Undergraduate Economics Association, the UC Berkeley Economics Department and faculty, or the University of California, Berkeley in general.