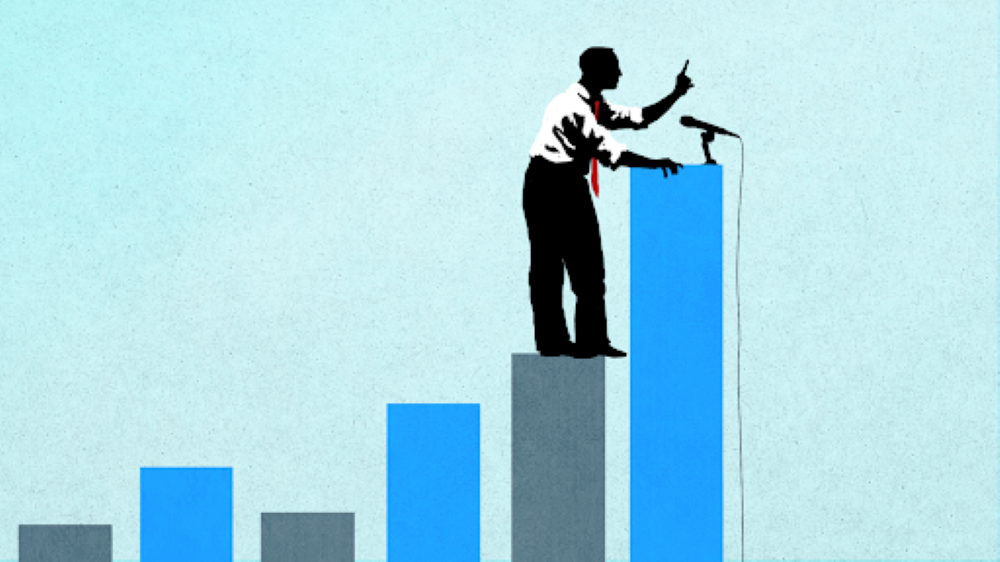
ANI BANERJEE – MARCH 4TH, 2020
EDITOR: SEAN O’CONNELL
Political polling for preferred candidates often seems to be a fool’s errand, even in the least controversial election years. Perhaps it is hindsight bias, but there are a myriad of polling issues lurking behind vague, generalized headlines like “Bernie Sanders Polling Well in Iowa.” Data journalism and various polls have become a fixture in election coverage in the US, but as the US becomes more diverse and more polarized, biases in sampling have led a majority of American to distrust election polls.
One of the primary flaws in current, large-scale election polling is overextension. Polling across too many states can lead to inaccuracies, so focusing on six to eight states often provides better results. Focused polls have the advantage of providing demographic weighting – taking into account what demographics are most likely to pick up a phone or fill out a survey and how those demographics might vote depending on the state. Furthermore, without knowing the specifics of a state, it is difficult to account for those who merely have phone numbers or IP addresses in the state (or county for caucuses), but are not registered to vote in that state. Attention to detail is therefore key in election surveying, but attention in general is a finite resource, which is why most representative election surveys only focus on several specific states. However, the natural response upon hearing that information is to select go to survey swing states as survey candidates. After all, if you only get up to eight states with accuracy, you might as well pick the states known for their unpredictability.
The ever elusive and sought after swing voter, despite only making up 7% of all votes cast, tends to have a disproportionate say in the outcome of any given election, particularly in swing states. These states, which include Pennsylvania and Wisconsin, could go either way in major elections, making them some of the most important states to poll. Swing states are important precisely because they’re unpredictable, and they’re unpredictable because voters there often do not decide their candidate preference until the last minute. However, this indecisiveness makes the task of surveying swing states that much harder, especially in the final two weeks of an election cycle, when as many as one in seven voters may remain undecided over who to vote for.
Some polls try to circumvent this dilemma by attempting to forecast based on likeability. Stanford professor Morris Fiorina argues, however, that since 1952, “likeability” has had very little to do with candidate success rates. His definition of likeability was operationalized into a specific set of judgements including intelligence/stupidity, arrogance/humility, sincerity/insincerity, and honesty/dishonesty. However, there is no mention in Fiorina’s paper of how these values are weighted, or even how they had been derived respective of the values of the American public. While he polled the American public on what they liked and disliked in a political figure, Fiorina neglected to analyze his own poll, meaning survey sample bias could proliferate.
Furthermore, in theory, the values desired in a president, like strength and assertiveness, are the values least desired in women. The “dis-likability” of the increasing amount of female candidates may be what’s tipping the scales — especially when likability is conflated with values like humility. While 94% of the country says that they’d vote for a woman in a presidential election, that theory may not hold up when a female candidate becomes a real option. Women elected to office in the US are still often much more qualified than the men that they run against. There is no way to tell what groups polled are most subject to this line of thinking, however, and which simply do not like a candidate based on their policies. The presence of sampling bias means that one cannot draw a conclusion about the American public’s opinions on issues based on their opinion of a candidate — especially when social movements like feminism have become so intrinsically tied to the Democratic party, rather than the Republican.
Therefore, to determine what issues are most important, a well-designed poll remains a necessity. Especially when it comes to issues like immigration, the problem is that the design of the poll matters quite a lot. If there is no Spanish — or Tagalog, Punjabi, or Cantonese — translation of a poll, how good of a representative sample will you create? If you only have online polls, can you accurately assess the urban-rural divide on issues?
The gold standard for polling used to be telephone-based surveys, although even phone errors were still fraught with large error bounds. The 1936 Literary Digest poll remains one memorable touchstone of a modern polling fiasco. It boasted a sampling error of 19% and predicted the presidential election in favor of Alfred Landon (R) over Franklin D. Roosevelt (D) despite having surveyed 10 million US voters. Nearly all of that sampling error in that case was the result of sample bias. Since the magazine had mailed their mock ballots to anyone on a telephone directory or club memberships, guaranteeing a bias towards the upper-middle class in an election that took place in the middle of the Great Depression. With telephone surveys, and surveys in general, a large sample size does not necessarily save one from a bad sampling method. Furthermore, as Democrats and Republicans are increasingly geographically isolated from each other, these issues in methods of aggregating addresses can come back to haunt pollsters.
But let’s be honest: when was the last time you answered a call from an unknown number? Response rates for phone polls have dropped from 36% in 1997 to 6% in 2019, as robocalls and filtering technology has proliferated. This means that most pollsters have had to turn to the internet, which has its own built in sample biases, namely that 10% of Americans don’t even use the internet, and there are clear demographic trends in who isn’t online. Online polls must therefore account for the fact that they miss a large percentage of older, low-income, and rural voters, and thereby weight demographics accordingly.
The realm of internet polling can be divided into two common types. The first option is the opt-in survey. It is both the least expensive and most commonly used type. The problems with this type of survey are exactly what you would imagine: the people who opt in are naturally going to self-select in certain directions, and these surveys face challenges in screening survey-takers. Depending on the websites a person frequents, how likely they are to have a poll advertised to them, and just the sort of personality that agrees to take online political polls, an opt-in poll can select for all sorts of confounding variables accidentally.
The second kind of poll has gotten more recent attention than opt-in surveys; they are probability-based online panels (PBOPs). You may have heard of a few of these PBOPs including: the Pew Research’s American Trends Panel and RAND Corporation’s American Life Panel. Both panels use this relatively new form of online polling.
Interestingly enough, one of these probability based online panels, Ipsos KnowledgePanel, has had global success through the populist wave in the last few years. In 2017, after unprecedented populist surges in France and the UK started sowing doubt in the accuracy of polls, Ipsos accurately predicted the results of the Dutch general election where the incumbent right-wing VVD party maintained their spot. They were also the second most accurate poll in India in 2019, even with the difficulties of exit polling in India. Their methods are mostly aimed at accounting for sampling biases and the demographic weighting explained above.
A Nature study assures us that despite a few spectacular left-field headlines in the past 5 years, polling in the US has grown more accurate than ever. Part of this may be the changing approach to internet polls, the way Ipsos compensates for sampling biases as those biases become more understood and accounted for. However, while the numbers are getting more and more accurate, the headlines are not.
The simplest reason for why polling is so inaccurate at predicting results has almost nothing to do with the polls themselves. Rather, pollsters are locked in an arms race with political polarization. The error must get smaller and smaller to keep up with the razor thin margins that candidates win by, which means that, in many cases, even the average error of 2 percentage points won’t be small enough. Elections are decided by narrower and narrower voting margins, which means that catching and understanding sampling bias becomes more and more important — especially as the deep political polarization in the US affects more and more of every-day life. It does not matter if a survey manages to accurately forecast 95% of all voter demographics if it neglects that the remaining 5% is where the deciding vote was cast.
Featured Image Source: Share.America.gov
Disclaimer: The views published in this journal are those of the individual authors or speakers and do not necessarily reflect the position or policy of Berkeley Economic Review staff, the Undergraduate Economics Association, the UC Berkeley Economics Department and faculty, or the University of California, Berkeley in general.